Health
Machine learning identifies early predictors of type 1 diabetes
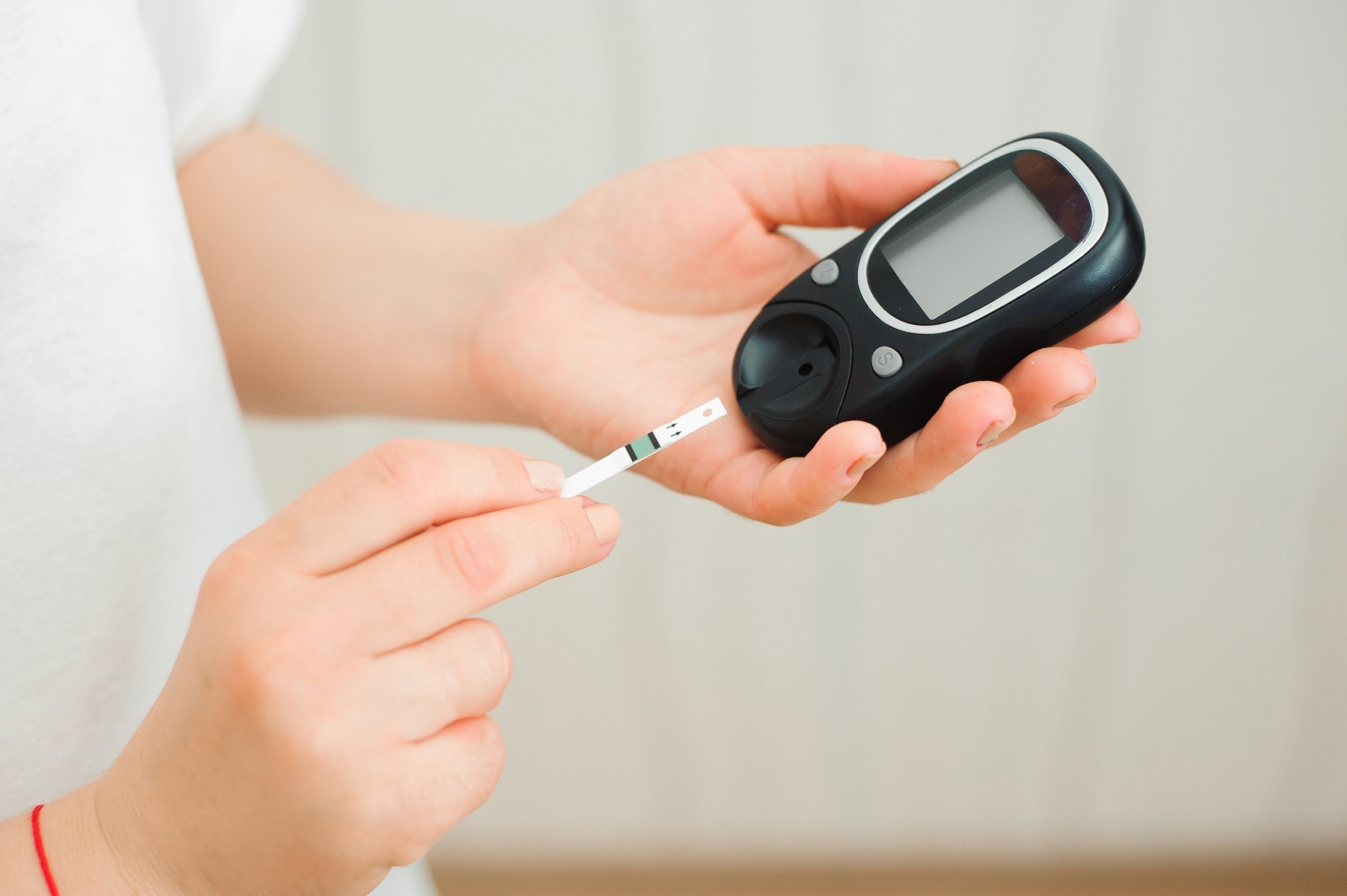
A recent study published in the journal cell report medicine Scientists used plasma protein proteomics to identify proteins associated with the development of type 1 diabetes, according to the Journal.
More than 2,250 samples from 184 participants yielded 376 regulatory proteins identified using machine learning analysis predictive of autoimmunity that precedes type 1 diabetes.
These results provide insight into the pathways altered during the development of type 1 diabetes, allowing disease to be predicted 6 months before onset.
study: Plasma protein biomarkers predict the development of persistent autoantibodies and type 1 diabetes 6 months before the onset of autoimmunity. Image credit: OleksandrNagaiets/Shutterstock.com
What is type 1 diabetes?
Type 1 diabetes (T1D) is an autoimmune disease that affects an estimated 20 million people worldwide, reducing their life expectancy by 11 years. It is characterized by the body’s rejection and destruction of β-cells due to the development of autoantibodies against an individual’s islet proteins, a process called ‘seroconversion’. A cure for this condition does not yet exist.
β-cells are responsible for insulin production, and their disruption leads to many diseases such as blindness, renal failure, and cardiovascular disease. So far, the triggers and mechanisms of T1D are poorly understood.
Recent programs, including the Environmental Determinants of Diabetes of the Young (TEDDY) study, have been launched to elucidate T1D and enable future therapeutic interventions.
These programs have identified plasma proteomics as a viable means to identify biomarkers associated with T1D, thereby gaining insight into the genetic and environmental determinants of disease.
Analysis of these proteins may improve the predictive power of researchers and, in the future, provide healthcare workers with a viable avenue to treat T1D. Unfortunately, many previous studies have failed to systematically validate study participants, leading to confusion in the interpretation of results.
About research
In this study, researchers conducted a nested case-control study of individuals in the TEDDY cohort. The two-phase study was divided into a discovery phase followed by a validation phase.
In the discovery phase, 184 randomly selected donors (92 samples + 92 controls) aged 0–6 years each provided 2,252 plasma samples collected at multiple time points over 18 months. These samples were sequenced using mass spectrometry and the resulting proteome was analyzed to identify the 14 most abundant proteins in each sample.
The validation phase consisted of 990 donors specifically selected based on biomarkers, genetic and demographic characteristics. The researchers developed and implemented a quality control analysis on real-time (QC-ART) system to ensure the quality of data collection and automated data management over the 18-month study.
Thus, 36,252 peptides were identified from 1,720 proteins, of which the 376 most frequently repeated proteins with the highest coefficient of variance were used for statistical analysis.
The researchers ultimately utilized machine learning (ML) models to predict phenotypes based on the 376 proteins identified in phases 1 and 2.
The model specifically tested whether the identified proteins could serve as biomarkers to predict whether a donor would remain in the islet autoimmune (IA) stage or progress to T1D. We ran 100 bootstrap iterations of these models and used logistic regression with a lasso penalty to build and identify the best model.
research result
In this study, we identified 376 proteins associated with the spectrum of IA, from normoglycemia to full T1D development.
These proteins were overexpressed in coagulation and complement cascade-related processes known to co-occur with T1D-related nutrient digestion and absorption, inflammatory signaling, blood clotting, and cellular metabolism.
Proteins identified from donors aged 3-9 months were found to successfully predict the development of T1D by age 6 years. A change in protein composition before seroconversion was observed in the donor’s metabolic profile, and the ML model allowed him to predict T1D 6–12 months before disease onset.
This study identified and validated 83 biomarkers that could be used in future clinical studies to identify T1D in patients with a genetic predisposition to T1D.
We believe that evaluation of these promising predictive protein panels in other ongoing prospective studies of autoimmunity and T1D development in human cohorts may aid in prognostic and therapeutic development. . ”
A major limitation of this study was that all donors were from the TEDDY study cohort, ie, individuals with a genetic predisposition to T1D and of American and European descent. Further studies involving people from more diverse geographic areas and those without a family history of T1D would help improve the robustness of these results.
Conclusion
Using thousands of TEDDY donor samples, researchers identified 376 proteins associated with future development of type 1 diabetes.
Machine learning models use these proteins to determine whether individuals with different permutations of these proteins remain carriers of T1D or develop autoimmune disease up to 6 months before disease onset. seroconversion may be accurately predicted.
Of the identified proteins, 83 have been termed ‘biomarkers’ and could be used in future clinical and scientific trials. This study is a robustly validated first step in understanding the underlying genetic mechanisms and environmental triggers of T1D.
This lays the groundwork for future studies that build on more geographically diverse samples. Ultimately, this study may pave the way for previously unavailable therapeutic interventions for this widespread condition.
Sources 2/ https://www.news-medical.net/news/20230703/Cutting-edge-research-machine-learning-identifies-early-predictors-of-type-1-diabetes.aspx The mention sources can contact us to remove/changing this article |
What Are The Main Benefits Of Comparing Car Insurance Quotes Online
LOS ANGELES, CA / ACCESSWIRE / June 24, 2020, / Compare-autoinsurance.Org has launched a new blog post that presents the main benefits of comparing multiple car insurance quotes. For more info and free online quotes, please visit https://compare-autoinsurance.Org/the-advantages-of-comparing-prices-with-car-insurance-quotes-online/ The modern society has numerous technological advantages. One important advantage is the speed at which information is sent and received. With the help of the internet, the shopping habits of many persons have drastically changed. The car insurance industry hasn't remained untouched by these changes. On the internet, drivers can compare insurance prices and find out which sellers have the best offers. View photos The advantages of comparing online car insurance quotes are the following: Online quotes can be obtained from anywhere and at any time. Unlike physical insurance agencies, websites don't have a specific schedule and they are available at any time. Drivers that have busy working schedules, can compare quotes from anywhere and at any time, even at midnight. Multiple choices. Almost all insurance providers, no matter if they are well-known brands or just local insurers, have an online presence. Online quotes will allow policyholders the chance to discover multiple insurance companies and check their prices. Drivers are no longer required to get quotes from just a few known insurance companies. Also, local and regional insurers can provide lower insurance rates for the same services. Accurate insurance estimates. Online quotes can only be accurate if the customers provide accurate and real info about their car models and driving history. Lying about past driving incidents can make the price estimates to be lower, but when dealing with an insurance company lying to them is useless. Usually, insurance companies will do research about a potential customer before granting him coverage. Online quotes can be sorted easily. Although drivers are recommended to not choose a policy just based on its price, drivers can easily sort quotes by insurance price. Using brokerage websites will allow drivers to get quotes from multiple insurers, thus making the comparison faster and easier. For additional info, money-saving tips, and free car insurance quotes, visit https://compare-autoinsurance.Org/ Compare-autoinsurance.Org is an online provider of life, home, health, and auto insurance quotes. This website is unique because it does not simply stick to one kind of insurance provider, but brings the clients the best deals from many different online insurance carriers. In this way, clients have access to offers from multiple carriers all in one place: this website. On this site, customers have access to quotes for insurance plans from various agencies, such as local or nationwide agencies, brand names insurance companies, etc. "Online quotes can easily help drivers obtain better car insurance deals. All they have to do is to complete an online form with accurate and real info, then compare prices", said Russell Rabichev, Marketing Director of Internet Marketing Company. CONTACT: Company Name: Internet Marketing CompanyPerson for contact Name: Gurgu CPhone Number: (818) 359-3898Email: [email protected]: https://compare-autoinsurance.Org/ SOURCE: Compare-autoinsurance.Org View source version on accesswire.Com:https://www.Accesswire.Com/595055/What-Are-The-Main-Benefits-Of-Comparing-Car-Insurance-Quotes-Online View photos
to request, modification Contact us at Here or [email protected]