Health
AI innovation “Sturgeon” transforms classification of central nervous system malignancies
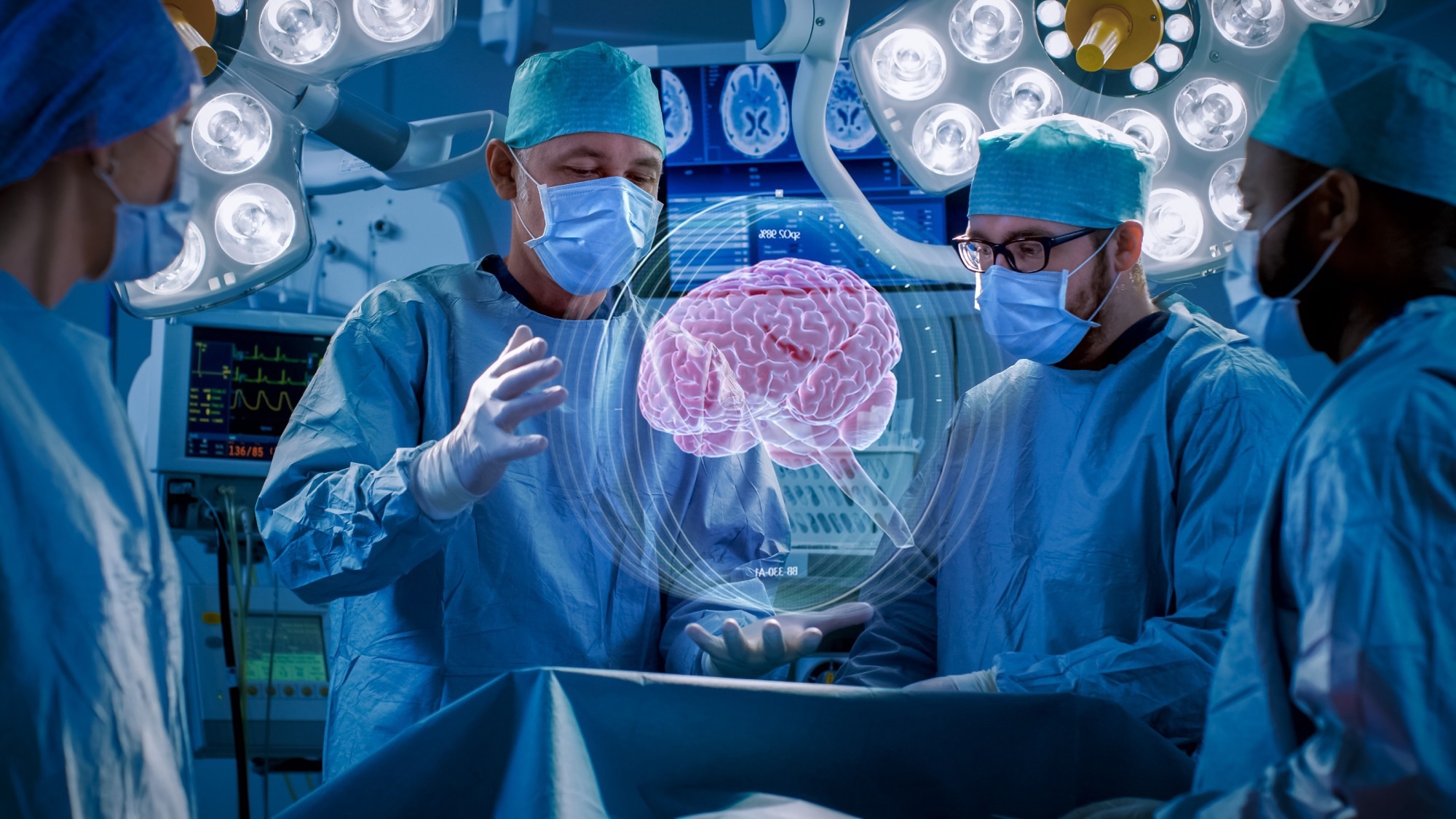
In a recent study published in Nature, researchers developed Sturgeon, a patient-agnostic transfer-learned neural network, to enable molecular subclassification of central nervous system (CNS) malignancies based on sparsity profiles.
Study: Ultra-fast deep-learned CNS tumour classification during surgery. Image Credit: Gorodenkoff/Shutterstock.com
Background
CNS tumors necessitate surgery and must be carefully resected. Current approaches, such as preoperative imaging and intraoperative histological analysis, might be incorrect.
Although rapid nanopore sequencing can aid in obtaining a sparse methylation profile during surgery, categorization remains difficult due to limited data and reference samples.
About the study
In the present study, researchers designed the Sturgeon machine learning classifier for pediatric and adult CNS tumor categorization, which may be utilized to improve surgical decision-making.
The researchers used nanopore sequencing data to create a model for predicting CNS tumor resection. The dataset was divided into four folds for submodel training, validation, and score calibration, including 2,801 reference labeled methylation profiles from CNS tumor and normal tissue samples.
The Sturgeon neural network was trained using a curriculum learning method, beginning with simple simulations and progressing to more difficult ones.
The network was trained using simulations ranging from 0.6% to 14% sparsity and fine-tuned with simulations with sparsity ranging from 0.6% to 6.3%. The classifier was fine-tuned for 3,000 epochs at a rate of 104 and validated on 50 batches (including 12,800 samples) for every 2,000 training batches by computing sensitivity and mean loss.
Samples were categorized using four submodels during inference, and the scores from the highest confidence level submodel were used for the final classification. The researchers adjusted the sequencing sparsity ranges to guarantee an equal distribution of simulated sequencing times.
The class balance was revised after each epoch by increasing class upsampling and/or simulation durations for which the machine learning model performed poorly. The temperature scaling method was used for model calibration.
The researchers generated 500 simulated samples from the reference dataset in the 0.6% to 14% sparsity range. To investigate Sturgeon’s resilience further, extra-realistic nanopore sequencing data was generated by randomizing the order of the sequenced reads.
The model was tested using 94 pediatric methylation profiles taken from people who had CNS tumor excision surgery at the Princess Máxima Center for Pediatric Oncology to assess its robustness to impure materials.
Results
Sturgeon provided a correct diagnosis in 45 of 50 retrospectively sequenced samples within 40 minutes of commencing sequencing. It was effective in real-time during 25 procedures, with a diagnostic turnaround time of less than 90 minutes. Of these, 18 (72%) were right, while seven did not meet the acceptable confidence level.
Sturgeon’s performance is directly proportional to the depth of the sequencing, with 0.6% to 4% of the 450K CpG regions covered during the first 50 minutes of simulated sequencing. Temperature scaling reduced the overall anticipated calibration error (ECE) in the test set from 0.025 to 0.002.
Sturgeon was identified properly (at the cut-off of 0.8) in 95% of instances with a definite diagnosis (32,412 of 34,000 sample simulations) in 25 minutes of sequencing simulations.
Using the 0.95 criterion, 86% of simulated samples (29,316 from 34,000) were properly identified. Performance increased somewhat after 50 minutes of sequencing simulations, with 97% (n=33,020) of model simulations attaining an accurate diagnosis with a confidence level of 0.80 and 91% with a 0.95 score.
Sturgeon was the first to transfer the computationally difficult model training, validation, and calibration process outside of the surgical time frame, resulting in well-tested, highly accurate one-size-fits-all classification models.
The classifier may be used in federated learning scenarios, with turnaround times of 1.5 hours for most samples, consistent with the surgical timeframe. Sturgeon could only perform successfully in samples with a large enough representation in the training data.
A greater proportion of admixed control readings degrades performance by increasing the number of situations where the classifier fails to obtain a confident classification.
Conclusions
Based on the study findings, machine-learned diagnosis based on low-cost intraoperative sequencing might help neurosurgeons make decisions, perhaps reducing neurological comorbidity and avoiding future procedures.
Sturgeon, a deep learning system trained on simulated nanopore sequencing data, could effectively detect tumor types in most pediatric instances within 25 to 50 minutes of sequencing simulations. With only 45 minutes of sequencing, it properly categorized 72% of cancers (18 out of 25) at the subclass level.
Ultra-fast methylation sequencing has enormous potential in other domains, such as regular post-operative diagnostics, lowering turnaround times, and enabling use in peripheral and low-income institutions. However, the needed amount of tissue might be a constraint.
Sturgeon might be used with histological evaluation by a competent pathologist, combining histological and molecular findings for a more accurate intraoperative diagnosis. The model might be useful in guiding decision-making in difficult circumstances when the histology diagnosis is uncertain.
Sources 2/ https://www.news-medical.net/news/20231016/AI-innovation-Sturgeon-transforms-pediatric-brain-tumor-surgery.aspx The mention sources can contact us to remove/changing this article |
What Are The Main Benefits Of Comparing Car Insurance Quotes Online
LOS ANGELES, CA / ACCESSWIRE / June 24, 2020, / Compare-autoinsurance.Org has launched a new blog post that presents the main benefits of comparing multiple car insurance quotes. For more info and free online quotes, please visit https://compare-autoinsurance.Org/the-advantages-of-comparing-prices-with-car-insurance-quotes-online/ The modern society has numerous technological advantages. One important advantage is the speed at which information is sent and received. With the help of the internet, the shopping habits of many persons have drastically changed. The car insurance industry hasn't remained untouched by these changes. On the internet, drivers can compare insurance prices and find out which sellers have the best offers. View photos The advantages of comparing online car insurance quotes are the following: Online quotes can be obtained from anywhere and at any time. Unlike physical insurance agencies, websites don't have a specific schedule and they are available at any time. Drivers that have busy working schedules, can compare quotes from anywhere and at any time, even at midnight. Multiple choices. Almost all insurance providers, no matter if they are well-known brands or just local insurers, have an online presence. Online quotes will allow policyholders the chance to discover multiple insurance companies and check their prices. Drivers are no longer required to get quotes from just a few known insurance companies. Also, local and regional insurers can provide lower insurance rates for the same services. Accurate insurance estimates. Online quotes can only be accurate if the customers provide accurate and real info about their car models and driving history. Lying about past driving incidents can make the price estimates to be lower, but when dealing with an insurance company lying to them is useless. Usually, insurance companies will do research about a potential customer before granting him coverage. Online quotes can be sorted easily. Although drivers are recommended to not choose a policy just based on its price, drivers can easily sort quotes by insurance price. Using brokerage websites will allow drivers to get quotes from multiple insurers, thus making the comparison faster and easier. For additional info, money-saving tips, and free car insurance quotes, visit https://compare-autoinsurance.Org/ Compare-autoinsurance.Org is an online provider of life, home, health, and auto insurance quotes. This website is unique because it does not simply stick to one kind of insurance provider, but brings the clients the best deals from many different online insurance carriers. In this way, clients have access to offers from multiple carriers all in one place: this website. On this site, customers have access to quotes for insurance plans from various agencies, such as local or nationwide agencies, brand names insurance companies, etc. "Online quotes can easily help drivers obtain better car insurance deals. All they have to do is to complete an online form with accurate and real info, then compare prices", said Russell Rabichev, Marketing Director of Internet Marketing Company. CONTACT: Company Name: Internet Marketing CompanyPerson for contact Name: Gurgu CPhone Number: (818) 359-3898Email: [email protected]: https://compare-autoinsurance.Org/ SOURCE: Compare-autoinsurance.Org View source version on accesswire.Com:https://www.Accesswire.Com/595055/What-Are-The-Main-Benefits-Of-Comparing-Car-Insurance-Quotes-Online View photos
to request, modification Contact us at Here or [email protected]