Health
Dengue dynamics, predictions, and future increase under changing monsoon climate in India
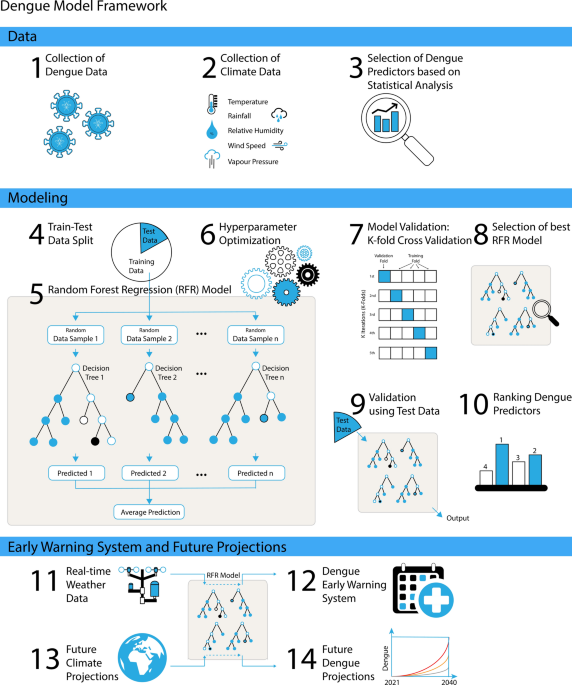
Dengue mortality over Pune shows a clear seasonality during the study period (2004–2015) (Fig. 2c). It is low from December to May but begins to rise after the onset of the summer monsoon rainfall in June and peaks in November. The maximum number of dengue mortalities reported over Pune during the study period was 81 in the year 2014.
(a) Geographical details of Pune district in Maharashtra, India. (b) Detailed view of Pune district highlighted in green. (c) Monthly cumulative (red bars) and mean (blue line) dengue mortalities over Pune from 2004 to 2015. Scatter plot (round markers) and linear fit (line plot) between normalized monthly mean values of (d) rainfall, (e) temperature, and (f) relative humidity on the x-axis and dengue mortalities over Pune on the y-axis. Data points are shown for zero lag in red and at the lag with maximum correlation in blue. Temperature, rainfall, and relative humidity show a statistically significant (p < 0.05) correlation of 0.38, 0.20, and 0.21 with dengue mortality at a lag of twenty weeks, eleven weeks, and six weeks, respectively.
Climatic factors affecting dengue mortality in Pune
By incorporating insights from the previous studies and establishing defined thresholds based on statistical analysis, we identified favorable windows of climatic conditions for increased dengue mortalities over Pune and devised a novel dengue metric for a preliminary statistical analysis. The novelty of our metric is that instead of looking into the direct relationship between climate and dengue, the number of days or weeks with favorable climatic conditions are considered here. Also, unlike existing studies examining the rainfall-dengue association, this metric employs three rainfall dependent variables—wet weeks, flush counts, and monsoon intraseasonal oscillations—to understand how rainfall patterns impact dengue mortality in Pune. The various climate-based predictors included in the metric are described in detail in the following sections (sections “Temperature” to “Humidity”).
Temperature
Mosquitoes, being poikilotherms, undergo fluctuations in their internal temperature depending on the surrounding environment. They face various risks, including desiccation (extreme drying), changes in metabolism, and reduced mobility due to thermal variations26. The mean temperature range of 27–35 °C is found to be optimal for increased dengue transmission considering several vector parameters like longevity, fecundity (the number of eggs deposited by each female vector), parity (the number of times a female has laid eggs), gonotrophic cycle (the time between a blood meal and oviposition), extrinsic incubation period (the time it takes for the virus to develop inside the mosquito), and intrinsic incubation period (time from human infection to symptom onset)12,27,28,29,30. However, it is important to verify if this temperature range favors dengue mortality in Pune as studies have shown that the optimal temperature for dengue transmission vary based on local climate conditions31,32. For instance, in Delhi, dengue transmission was positively correlated with higher temperatures, even in the range of 30–39 °C31. In contrast, the highest mean temperature recorded in Pune during the study period (2004–2015) was 35 °C, suggesting that the identified optimal temperature range (27–35 °C) is specific to Pune’s climate. As shown in Supplementary Fig. S1-a, we find a statistically significant positive correlation (r = 0.70, p < 0.05) between the annual dengue mortality and the number of days with optimal temperatures (27–35 °C) during the summer monsoon season (June to September) in Pune. This temperature window is specific to Pune and maybe different for other regions. Thus, rather than looking at the direct relationship between dengue and temperature, the number of days with favorable conditions for dengue vector and virus yields a more meaningful result.
Rainfall flushes and wet weeks
To better understand the rainfall-dengue relationship, we introduced rainfall-dependent variables, such as wet weeks, and rainfall flushes for Pune. Wet weeks are defined as weeks with a minimum of 0.5 mm and a maximum of 150 mm of rainfall, and when the weekly cumulative rains exceed 150 mm, such events are termed rainfall flushes. The current study reveals that weekly cumulative rains between 0.5 mm and 150 mm lead to an increase in dengue mortality (r = 0.85, p < 0.05) (Supplementary Fig. S1-b, Table 1), while rains above that threshold reduce dengue mortality (r = − 0.77, p < 0.05) in Pune through the flushing effect (Supplementary Fig. S1-c, Table 1). Rainfall thresholds that are used to define flushing can vary depending on the region33,34. Various weekly rainfall thresholds were examined to identify the one that exhibits the strongest correlation with dengue mortality in Pune. The current study recommends using a weekly threshold to define wet weeks and rainfall flushes, considering the cumulative effect of rainfall reaching the threshold within a week.
Monsoon intraseasonal oscillations
The Indian summer monsoon rainfall exhibits strong variability at intraseasonal timescales, known as monsoon intraseasonal oscillations, characterized by active (wet) and break (dry) phases of the monsoon35,36. Monsoon intraseasonal oscillations are obtained using bandpass filtering for the dominant intraseasonal timescale (30–90 days) (Fig. 3a)37,38,39. We consider active days as those with rainfall anomalies exceeding 0.5 standard deviations of the summer monsoon (June to September) rainfall anomaly of the baseline period from 1995–2015, while break days are defined as those with rainfall anomalies less than − 0.5 standard deviations. As demonstrated in Fig. 3b and Table 1, the years reporting high dengue mortality in Pune (2010, 2014, and 2015) are characterized by a lower number of active and break days. Conversely, the years with low dengue mortality (2008, 2011, and 2013) are characterized by a higher number of active and break days (r = − 0.74, p < 0.05). Currently, the India Meteorological Department provides extended-range forecasts with information on the active-break cycles of monsoon, 10–30 days in advance for the entire country. Utilizing these forecasts can offer additional lead time for dengue predictions. Thus, monsoon intraseasonal oscillations could serve as a valuable predictor of dengue with improved skills in ISMR predictions.
(a) Rainfall anomaly timeseries filtered for its intraseasonal oscillations during 2010–2012 (note that this is for a sample period, and the entire filtered time series is not shown here). Active (blue shaded) and break days (red shaded) are those with rainfall anomalies greater than 0.5 standard deviations (dashed red line) and less than –0.5 standard deviations, respectively, of the summer monsoon rainfall anomaly (June–September) from 1995–2015. (b) Count of active (blue bars), break days (red bars), and dengue mortality (black line) from 2004 to 2015 over Pune. The number of active and break days is lower in high dengue mortality years (2010, 2014, 2015) compared to other years with low dengue mortality.
The rainfall-dependent variables presented here highlight clear differences in the patterns of rainfall during high and low dengue years in Pune. As shown in Table 1, high dengue years in Pune have fewer rainfall flushes, more wet weeks, and fewer active-break days. An increased number of wet weeks, along with fewer rainfall flushes, indicates that rainfall is more evenly spread over time, reducing the likelihood of mosquito eggs and larvae being washed away. Fewer active-break days also suggest that the variability in monsoon rainfall is lower during high dengue years. Thus, years with high dengue mortality in Pune are associated with moderate rainfall distributed over time.
Conversely, low dengue years are linked to more rainfall flushes and fewer wet weeks (Table 1). Although these years have higher total rainfall, the rains are extreme, which can flush out mosquito eggs and larvae. Additionally, the increased number of active-break days suggests greater variability in rainfall during low dengue years, with more number of extreme rainfall events. This pattern contrasts with the moderate, evenly distributed rains that favor dengue transmission.
In summary, our findings suggest that it is not the cumulative amount of rainfall, but rather the pattern of rainfall, that plays a crucial role in influencing dengue transmission in Pune. Moderate rains spread over time creates favorable conditions for dengue, whereas extreme rainfall events reduce the dengue risk through their flushing effect.
June cumulative rains
Major rain-bearing months in Pune are from June to September, and more than 80% of dengue mortalities in the district occur after the onset of monsoon in June (Fig. 2c). Studies have shown that mosquito eggs can survive drying out for up to eight months, implying that eggs laid after the monsoon season in the preceding year may rely on the June rains of the current year for survival15,40. However, heavy rains in June can wash out those eggs and play an important role in reducing dengue-related mortalities for the entire year by controlling the vector population. We find a statistically significant negative association (r = − 0.66, p < 0.05) between annual dengue mortality and the June cumulative rains in the corresponding year in Pune during the study period (2004–2015) and is more evident in recent years with high dengue mortality (Supplementary Fig. S1-d). Conversely, we find no statistically significant correlations (p < 0.05) between cumulative rainfall in the other monsoon months and annual dengue mortality (Supplementary Fig. S7, Table S3).
However, it’s important to note that all monsoon months significantly contribute to mosquito breeding and dengue incidence and the other rainfall-dependent variables in the dengue metric, such as wet weeks and flush counts, are calculated based on rainfall throughout the entire year.
Humidity
Relative humidity is found to favor the percentage of hatching, survival rate, and biting frequency of adult dengue mosquitoes and allows the infected female mosquitoes to complete more than one replication cycle of the virus16,41. A minimum of 60% relative humidity is required for Ae. aegypti mosquitoes to survive because low humidity causes water to evaporate from the mosquito’s body and dries out their body fluids42. Also, the mosquitoes will have a short lifespan below 60% humidity and will not be able to transfer the virus from its stomach to salivary glands, which prevents them from becoming vectors43. Conversely, an increase in relative humidity beyond a specific threshold can result in a decrease in dengue incidence44,45. This is a critical environmental parameter for Pune where the humidity shows high variability across the seasons. According to our analysis, dengue mortality over Pune is high when relative humidity levels are between 60 and 78% during the summer monsoon season (June to September) (r = 0.72, p < 0.05). This range closely aligns with findings from a study in Delhi, India, where a relative humidity of 70–80% was identified as ideal for Ae. aegypti mosquitoes46. Additionally, studies from Guangzhou, China—another monsoonal region—reported similar upper thresholds of 76% and 79% for relative humidity favoring increased dengue incidences32,45. Like temperature and precipitation, relative humidity also exhibits a non-linear relationship with dengue (Supplementary Fig. S1-e).
In conclusion, our analysis of observed dengue mortality and meteorological variables from 2004 to 2015 in Pune demonstrates that moderate rainfall distributed over time contributes to an increase in dengue mortality, while heavy rains have the potential to reduce it through flushing effect. Furthermore, our findings indicate that years with higher dengue mortality rates in Pune are characterized by temperatures ranging from 27 °C to 35 °C and relative humidity levels between 60 and 78% during the Indian summer monsoon season. The dengue metric presented here is part of the preliminary statistical analysis aimed at understanding the climatic conditions that favor dengue mortality in Pune and is based on the basic linear correlation analysis. However, the climate-dengue associations are complex due to the non-linear and compound interactions between potential predictor variables. Therefore, we developed a machine learning model to better capture and analyze these intricate non-linear associations.
Dengue early warning system for Pune
Machine learning methods are found to be superior to traditional statistical methods in understanding and predicting the complex interactions between multiple variables47. To identify potential climatic variables that influence dengue incidence in Pune and to understand their cumulative effect, we used the machine learning technique of Random Forest Regression (RFR) on 12 years of observed dengue mortality and meteorological data (2004–2015) at weekly timescales. Random Forest is an ensemble learning method that combines the predictions of multiple decision trees to create a more robust and accurate model48. A combination of individual models like this can help to reduce overfitting and improve generalization.
Despite the availability of many advanced machine learning algorithms, RFR was selected for this study due to its favorable prediction performance in prior research49,50,51 and its relative ease of comprehension, making it suitable for researchers new to machine learning. RFR is highly flexible and can handle high-dimensional data with non-linear effects and numerous covariate interactions. Its built-in mechanism to rank significant predictors also provides valuable insights into the model predictions50. Predictor inputs used in the RFR model (dengue model from here) are weekly means of temperature, cumulative rainfall, relative humidity, and weekly count of active and break days. These weekly predictors were chosen from a larger group of variables based on statistical analyses and the improvement in model skill (Fig. 1, steps 2 and 3). The other derivative variables from the dengue metric (section “Climatic factors affecting dengue mortality in Pune”) were not used as additional individual predictors in the dengue model, as their inclusion did not enhance model skill. This is because RFR is a decision tree-based algorithm, capable of capturing the non-linear associations between predictors and the target52. It can identify the optimal climatic conditions for increased dengue risk using basic climate variables such as mean temperature, cumulative rainfall, and mean relative humidity, without the need to explicitly include derived variables like the count of days with optimal temperature, wet weeks, or flush counts. Although the dengue metric variables were not directly used in the model, they aid in interpreting the predictions and projections generated by the dengue model.
It is critical to incorporate the lag between favorable weather conditions and dengue mortality into the dengue model, as it accounts for multiple processes occurring over different time spans, from mosquito breeding to human host getting infected with the dengue virus. The mosquito’s lifecycle from an egg to an adult, takes about 8–10 days, while the extrinsic incubation period of the dengue virus inside an infected mosquito takes 5–33 days12. After infecting a human through a mosquito bite, the virus has an intrinsic incubation period of about 2–15 days in the human body53. A person typically develops viremia, a condition characterized by a high level of the dengue virus in the blood, four days after being bitten by an infected Ae. aegypti mosquito. Viremia can last up to 12 days but usually lasts around five days54. Studies report that the possible lag between hospital admission (after the onset of symptoms) and dengue mortality ranges from 3–15 days55,56. Altogether, from the availability of aquatic habitats for mosquito breeding to a dengue mortality, it takes approximately 1–3 months (8–73 days). In addition to this, the dengue vectors capable of transmitting dengue virus can survive for about 30 days21.
Similar to the methodology followed in the prior studies on dengue modeling using climatic variables, input variables are provided to the dengue model at the lags where variable-dengue correlations are strongest57,58. Using cross-correlation analysis, our study finds that dengue mortality over Pune (2004–2015) shows maximum correlation with mean temperature (r = 0.38, p < 0.05) and cumulative rainfall (r = 0.20, p < 0.05) at lags of 20 and 11 weeks, respectively (Fig. 2d,e). Relative humidity shows a statistically significant correlation with dengue mortality at a lag of six weeks (r = 0.21, p < 0.05), with a slightly stronger correlation at lag zero (r = 0.22, p < 0.05) (Fig. 2f). Though relative humidity at lag zero had a slightly stronger correlation with dengue mortality, relative humidity at a lag of six weeks was chosen for the dengue modeling due to its potential for longer-lead predictions. The dengue model achieved highest prediction skill by using temperature twenty weeks prior, rainfall eleven weeks prior, and relative humidity six weeks prior for predicting dengue mortality of the current week.
It is important to note that these linear correlation values are part of the preliminary statistical analysis aimed at identifying the lag in climate-dengue association (Fig. 2d–f) and do not represent the predictive power of each variable in forecasting dengue mortality. Given the non-linear and complex nature of climate-dengue interactions, we further developed a machine learning-based dengue model using the random forest regression algorithm to capture the intricate relationships between climatic variables and dengue.
We used the meteorological and dengue mortality data from 2005–2014 for training and the years 2004 and 2015 for testing the dengue model. At the end of the optimization of model parameters using RandomizedSearchCV and K-fold cross-validation (Fig. 1, steps 6 and 7), the model yields a good prediction skill with a statistically significant correlation coefficient of r = 0.77 (p < 0.05) and a low Normalized Root Mean Squared Error (NRMSE) score of 0.52 between the actual and predicted dengue mortalities during the test period, indicating that the model can effectively predict dengue mortality in Pune (Fig. 4a).
(a) Comparison of weekly cumulative dengue mortalities in Pune (x-axis) and the corresponding mortality predictions from the dengue model (y-axis) during the test period 2004 and 2015. The model shows good prediction skill, with a statistically significant correlation coefficient of r = 0.77 (p < 0.05) and a low normalized root mean squared error (NRMSE) of 0.52 between actual and predicted dengue mortalities. These results indicate that the model can effectively predict dengue mortality in Pune. (b) Relative importance of each predictor variable in predicting the dengue mortality in Pune (variable importance). The variable importance of temperature, rainfall, relative humidity, active days, and break days are 41%, 29%, 20%, 7%, and 3%, respectively. Monsoon rainfall and its variability together account for 39% of the variable importance.
To understand the role of different weather variables in predicting Pune’s dengue mortality, we utilized the variable importance feature of Random Forest algorithms. Variable importance refers to the degree of association between a given predictor variable and the model predictions. From the observed climate and dengue mortality data, the RFR model finds that mean temperature and relative humidity has a variable importance of 41% and 20%, respectively, in predicting dengue mortality over Pune (Fig. 4b). Rainfall and its variability together account for 39% of variable importance, with cumulative rainfall contributing 29% and monsoon intraseasonal variability (active-break days) contributing 10%. The variable importance is directly linked to the model skill, indicating that 41% of the model’s prediction skill is driven by mean temperature and 29% by cumulative rainfall.
Dengue future projections for Pune
Monsoon conditions, particularly rainfall patterns, are projected to change in the coming years and decades over India59. This may have significant implications for Pune since the dengue mortality in the region exhibits a strong dependence on the rainfall pattern. The dengue model developed based on the observed climate-dengue associations in Pune is used in conjunction with climate change projections from the Coupled Model Intercomparison Project phase 6 (CMIP6) models for depicting future projections of dengue mortality in the district (Fig. 5a). Dengue mortality in Pune is projected under three distinct Shared Socioeconomic Pathways (SSPs), representing different levels of socioeconomic development and greenhouse gas emissions. SSP1, SSP2 and SSP5 correspond to low, intermediate, and high emissions, respectively. Eight CMIP6 models that best represent the interannual variability in the intraseasonal oscillations of the Indian summer monsoon and spatial distribution of the mean precipitation over India are selected for assessing the future projections of climate and dengue in Pune (Supplementary Table S1). All climatic predictors of the dengue in Pune were computed from the CMIP6 model outputs and provided as inputs into the dengue model at optimal lags to obtain the dengue future projections for the region.
(a) The dengue mortality predictions for Pune, based on the dengue model developed for Pune (1950–2100) for the historical (1950–2014) and the future emission pathways (2015–2100, SSP1, SSP2, and SSP5) relative to the reference period of 1995–2014 (light-grey bar). The multi-model ensemble means were computed using a 20-year smoothed time series. Shaded regions in the time series indicate the range of uncertainty or variability, represented by one standard deviation of dengue mortality projections across selected models. (b) Projected percentage changes in dengue mortalities over Pune in the near future (2021–2040), mid-century (2041–2060), and late century (2081–2100) emission pathways, relative to the reference period (1995–2014). Changes in average global surface temperature, relative to the pre-industrial period (1850–1900), are indicated in red font within the bars corresponding to the three emission pathways for near future, mid-century, and late century.
According to the future projections obtained from the dengue model, dengue mortalities over Pune are projected to rise in the future under all emission pathways (SSPs) (Fig. 5a,b). In the near future (2020–40), regardless of the emission pathways, a 12–13% increase in dengue mortalities is expected relative to the reference period (1995–2014). Whereas in the mid-century (2040–60), dengue mortality over Pune is projected to increase by 25% under SSP2 and 40% under SSP5 relative to the reference period (1995–2014) (Fig. 5b). This increase is amplified in the late century (2081–2100), with projections of up to a 112% increase in dengue mortality by 2100 under SSP5. In the late century, the percentage increase in dengue mortality under SSP1 and SSP2 is 30% and 58%, respectively (Fig. 5b). The first virologically proven dengue epidemic in India occurred in Calcutta and the Eastern Coast of India in 1963–196460,61, and hence the future projections are considered from 1950 onwards.
In Fig. 5b, the changes in average global surface temperature compared to the pre-industrial era (1850–1900) are indicated in red font within the bars corresponding to SSP1, SSP2, and SSP5 pathways for the near future, mid-century, and late century time periods. If future emissions can be controlled to keep the global average surface temperature changes below 1.5 °C, the anticipated rise in dengue mortalities relative to the reference period will be below 13%. However, if emissions continue to increase and temperatures exceed the 1.5 °C threshold, the corresponding increase in dengue mortality ranges from 23–40% (Fig. 5b). Alternatively, when the global surface temperature crosses the 2 °C threshold, dengue mortalities are projected to increase by 40–112% relative to the reference period.
Climatic changes leading to the projected increase in dengue in Pune
To understand the climatic changes leading to the projected increase in dengue mortalities over Pune (Fig. 5a,b), we analyzed the future projections of environmental predictors of dengue in Pune. The future projections from the selected CMIP6 models show that all environmental predictors of dengue are expected to increase in the future (relative to historical levels), with greenhouse gas emissions and socio-economic pathways having a particularly pronounced effect on future projections towards the late century (2081–2100) (Supplementary Fig. S3). In the near-term, the meteorological variables show little change relative to their respective reference period (1995–2014) values. However, by the mid-century, the mean temperature is likely to increase by 1–1.5 °C, cumulative rainfall by 23–27 cm, relative humidity by 0.4–1%, and the number of active-break days by 1–3 days from their corresponding reference period values (Supplementary Table S2). In the far-future, selected CMIP6 models indicate an increase in mean temperature, cumulative rainfall, mean relative humidity, count of active and break days in the range of 1.2–3.5 °C, 28–65 cm, 0.2–1.6%, and 2–5 days, respectively, under the three emission pathways considered in this study. The lower limit in the ranges corresponds to the low emission scenario (SSP1), while the maximum changes are projected under the higher emission scenario (SSP5).
The future trends (2015–2100) in the climatic predictors of dengue mortality in Pune is shown in Table 2. Both temperature and rainfall demonstrate a statistically significant increasing trend across all emission pathways. The count of active-break days shows a statistically significant increasing trend only under SSP2 and SSP5. Additionally, relative humidity exhibits an increasing trend only in the high-emission scenario, SSP5.
Even though all meteorological predictors of dengue mortality in Pune are projected to increase in the future, their collective impact on dengue mortality cannot be determined through linear analysis alone. The dengue model was used to gain insights into the combined effect of projected changes in dengue predictors on dengue mortality in Pune. To isolate the impact of these trends in the predictor variables on dengue mortality projections, we performed experiments detrending the climatic predictors in the dengue model. In these experiments, each predictor variable was detrended individually while keeping the other predictors unchanged. The results of these experiments are presented in Supplementary Figs. S4 and S5. According to these experiments, the trend in the mean temperature has a significant influence, contributing to a 12–22% increase in dengue mortality across low-to-high emission scenarios (Supplementary Fig. S5). In contrast, the trend in rainfall offsets dengue mortality by 2–4% (Supplementary Fig. S5). The percentage changes are calculated for the detrending experiments relative to the original dengue mortality projections. The trends in other variables have little effect on the dengue mortality in Pune.
Our analysis of observed meteorological variables and reported dengue mortalities from 2004 to 2015 in Pune (presented in section “Climatic factors affecting dengue mortality in Pune”) reveals a complex relationship between rainfall patterns and dengue mortality. Specifically, we found that moderate rains spread in time lead to increased dengue mortality over Pune, whereas heavy rains reduce it through the flushing effect (Table 1). To further investigate if the dengue model is picking up this relationship while giving future projections, we performed an experiment in which we modified the rainfall pattern in the selected CMIP6 model simulations by eliminating all extreme rainfall events and testing the impact on dengue mortality. Here we considered extreme rainfall as those with values greater than the 90th percentile of the reference period rainfall time series (1995 and 2014). Our results show that the removal of extreme rainfall events lead to an increase in dengue mortality by about 3–4% (refer to Supplementary Figs. S4b, d, f, and S5). Thus, future extreme rainfall events can be expected to offset the increase in dengue by 3–4%. This result is consistent with our findings from observational data, which suggest that extreme rainfall tends to reduce dengue transmission, while moderate rainfall is likely to increase dengue incidence.
In summary, our study reveals that the projected increase in dengue mortality over Pune is primarily driven by the increase in temperature and changes in rainfall patterns. These results underscore the importance of a comprehensive and multivariate approach to assessing the risks associated with dengue transmission. Furthermore, it emphasizes the importance of reducing greenhouse gas emissions to mitigate the harmful impact of climate change on public health.
Sources 2/ https://www.nature.com/articles/s41598-025-85437-w The mention sources can contact us to remove/changing this article |
What Are The Main Benefits Of Comparing Car Insurance Quotes Online
LOS ANGELES, CA / ACCESSWIRE / June 24, 2020, / Compare-autoinsurance.Org has launched a new blog post that presents the main benefits of comparing multiple car insurance quotes. For more info and free online quotes, please visit https://compare-autoinsurance.Org/the-advantages-of-comparing-prices-with-car-insurance-quotes-online/ The modern society has numerous technological advantages. One important advantage is the speed at which information is sent and received. With the help of the internet, the shopping habits of many persons have drastically changed. The car insurance industry hasn't remained untouched by these changes. On the internet, drivers can compare insurance prices and find out which sellers have the best offers. View photos The advantages of comparing online car insurance quotes are the following: Online quotes can be obtained from anywhere and at any time. Unlike physical insurance agencies, websites don't have a specific schedule and they are available at any time. Drivers that have busy working schedules, can compare quotes from anywhere and at any time, even at midnight. Multiple choices. Almost all insurance providers, no matter if they are well-known brands or just local insurers, have an online presence. Online quotes will allow policyholders the chance to discover multiple insurance companies and check their prices. Drivers are no longer required to get quotes from just a few known insurance companies. Also, local and regional insurers can provide lower insurance rates for the same services. Accurate insurance estimates. Online quotes can only be accurate if the customers provide accurate and real info about their car models and driving history. Lying about past driving incidents can make the price estimates to be lower, but when dealing with an insurance company lying to them is useless. Usually, insurance companies will do research about a potential customer before granting him coverage. Online quotes can be sorted easily. Although drivers are recommended to not choose a policy just based on its price, drivers can easily sort quotes by insurance price. Using brokerage websites will allow drivers to get quotes from multiple insurers, thus making the comparison faster and easier. For additional info, money-saving tips, and free car insurance quotes, visit https://compare-autoinsurance.Org/ Compare-autoinsurance.Org is an online provider of life, home, health, and auto insurance quotes. This website is unique because it does not simply stick to one kind of insurance provider, but brings the clients the best deals from many different online insurance carriers. In this way, clients have access to offers from multiple carriers all in one place: this website. On this site, customers have access to quotes for insurance plans from various agencies, such as local or nationwide agencies, brand names insurance companies, etc. "Online quotes can easily help drivers obtain better car insurance deals. All they have to do is to complete an online form with accurate and real info, then compare prices", said Russell Rabichev, Marketing Director of Internet Marketing Company. CONTACT: Company Name: Internet Marketing CompanyPerson for contact Name: Gurgu CPhone Number: (818) 359-3898Email: [email protected]: https://compare-autoinsurance.Org/ SOURCE: Compare-autoinsurance.Org View source version on accesswire.Com:https://www.Accesswire.Com/595055/What-Are-The-Main-Benefits-Of-Comparing-Car-Insurance-Quotes-Online View photos
to request, modification Contact us at Here or [email protected]