Health
Machine learning applications for cancer diagnosis, treatment, and prognosis
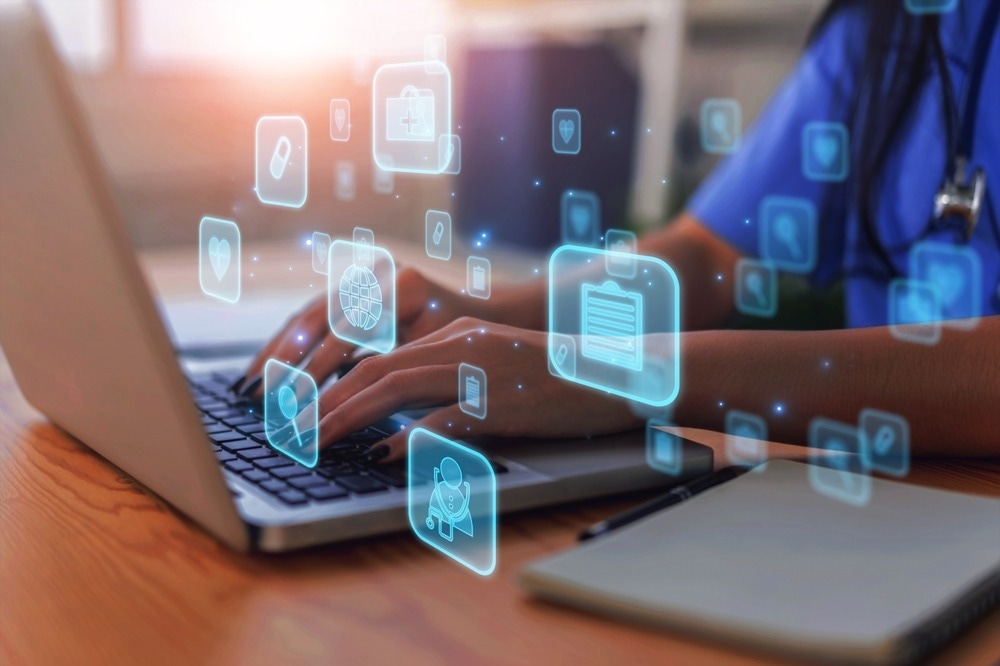
Machine learning (ML) models are increasingly being used in clinical oncology for cancer diagnosis, outcome prediction, and to inform tumor treatment plans. Early detection and rapid treatment of cancer will be revolutionized by the rapid and accurate analysis of tissue radiological and pathological images using ML algorithms to improve the chances of survival and the quality of care provided to cancer patients. can be improved.
In a recent review published in the journal cellStanford University researchers review the application of ML in cancer diagnosis, treatment, and improving prognosis.
study: From Patterns to Patients: Advances in Clinical Machine Learning for Cancer Diagnosis, Prognosis, and Treatment. Image credit: Have a nice day Photography / Shutterstock.com
Common ML models in oncology
ML models are based on supervised learning and each data point has an associated label. Commonly used ML models include random forest models, support vector machines (SVM), regression models, neural networks, recurrent neural network (RNN) models, convolutional neural network (CNN) models, transformers, graph neural networks ( GNN) model, etc.
A random forest model does its inference by building a decision tree based on a few binary decisions on the input. The SVM model provides a line or multidimensional hyperplane of tumor features by separating the various data point classes from the maximum possible boundaries between the data classes. Regression models linearly combine inputs to estimate continuous and binary labels by linear and logistic regression, respectively.
A neural network consists of multiple layers of neurons that iteratively compute a linear assimilation of an input variable, followed by a nonlinear function, to estimate an outcome such as cancer probability. RNN models process sequential information such as genome sequences or image series by applying a similar layer of neural networks to all objects present in the sequence and remembering the observed objects.
CNN models apply neural patches or “filters” that scan images and identify patterns. The first layer detects low-level features such as edges, and subsequent layers detect high-level features such as tumor cell morphology. Transformers analyze sequential information by repeatedly applying attention operations to compare the sequential with other components and update the internal sequence representation.
GNN models evaluate graph-structured information, such as cell-to-cell interaction graphs. A model encodes the basic characteristics of nodes and edges in a graph. This information is passed by the layers of the neural network as they traverse the ML graph to update the corresponding representations.
The representation is used to infer graph labels. All common model classes have specific architectures and differ in the size and number of neural network layers.
ML for cancer diagnosis, prognosis, and treatment
All patients will be imaged using pathological, radiological, and other imaging modalities. High-resolution images are segmented into image tiles that span the entire image or just regions of interest (ROI) for processing by ML models. A CNN model processes image tiles and produces pixel-level or tile-level predictions. Heatmaps predict where tumors are likely to arise.
Additionally, tile-level outputs are aggregated into a single output using formulas or ML models like RNNs. A final estimation component, such as a neural network, uses the tiled output integrated into label predictions that are evaluated using metrics. Labels can come from a variety of sources, such as biopsy or radiology, and can be of several types, including binary labels for tumor classification and real-valued labels for tumor regression.
Radiographic imaging is used to detect potentially malignant lesions during routine screening or when symptomatic. If radiographic images suggest cancer, a biopsy is obtained and the diagnosis is confirmed by analyzing histopathological images. Radiological and pathological images are also used for prognostic assessment and selection of the most appropriate treatment.
Common molecular datasets that can be obtained by single-cell transcriptomics and spatial proteomics, bulk ribonucleic acid (RNA) sequencing of tumor biopsies, and whole-genome sequencing include circulating cell-free deoxyribonucleic acid (cfDNA), fragmentmics, Epigenetic modifications, and the state of DNA methylation. These datasets are incorporated into SVMs, elastic net models, random forest classifiers, and Bayesian models for selecting types of cancer treatments and predicting responses to them.
Random forest classifiers can identify tumor origins using consecutively occurring cytosine and guanine (CpG) DNA sites and microRNAs (miRNAs). Cell-type-specific gene profiles can be inferred using ML without physically separating cells. GNN can predict cancer outcome from spatial proteomics of head and neck cancer.
Elastic net models can predict response to immunotherapy from DNA fragment mix profiles. Data considerations for ML include signal-to-noise ratio, sparsity, dimensionality, and feature selection.
Several ML medical devices for cancer are approved by the U.S. Food and Drug Administration (FDA) and Clinical Laboratory Improvement Amendments (CLIA) for breast mammography, gastrointestinal endoscopy, and prostate cancer by magnetic resonance imaging (MRI). Approved for use in the detection of SVM and lung cancer from chest radiographs and computed tomography (CT) using CNN. ML devices have also been used to detect ovarian cancer.
Conclusion
The current review focuses on ML models used in oncology and routine ML pipelines for image-based diagnosis, treatment, and prognosis estimation from molecular features of liquid and solid tissue samples. .
ML prediction stratifies cancer risk, assesses risk factors such as breast density for breast cancer, detects tumor cells, aids in treatment selection, and analyzes cancer subtype, mutational status, tumor metastasis, Cancer outcomes can be predicted by identifying microsatellite instability, patient survival, and response. For radiotherapy, chemotherapy and immunotherapy.
Journal reference:
- Swanson, K., Wu, E., Zhang, A., others(2023). From Patterns to Patients: Advances in Clinical Machine Learning for Cancer Diagnosis, Prognosis, and Treatment. cell. doi:10.1016/j.cell.2023.01.035
Sources 2/ https://www.news-medical.net/news/20230319/Machine-learning-applications-for-the-diagnosis-treatment-and-prognosis-of-cancer.aspx The mention sources can contact us to remove/changing this article |
What Are The Main Benefits Of Comparing Car Insurance Quotes Online
LOS ANGELES, CA / ACCESSWIRE / June 24, 2020, / Compare-autoinsurance.Org has launched a new blog post that presents the main benefits of comparing multiple car insurance quotes. For more info and free online quotes, please visit https://compare-autoinsurance.Org/the-advantages-of-comparing-prices-with-car-insurance-quotes-online/ The modern society has numerous technological advantages. One important advantage is the speed at which information is sent and received. With the help of the internet, the shopping habits of many persons have drastically changed. The car insurance industry hasn't remained untouched by these changes. On the internet, drivers can compare insurance prices and find out which sellers have the best offers. View photos The advantages of comparing online car insurance quotes are the following: Online quotes can be obtained from anywhere and at any time. Unlike physical insurance agencies, websites don't have a specific schedule and they are available at any time. Drivers that have busy working schedules, can compare quotes from anywhere and at any time, even at midnight. Multiple choices. Almost all insurance providers, no matter if they are well-known brands or just local insurers, have an online presence. Online quotes will allow policyholders the chance to discover multiple insurance companies and check their prices. Drivers are no longer required to get quotes from just a few known insurance companies. Also, local and regional insurers can provide lower insurance rates for the same services. Accurate insurance estimates. Online quotes can only be accurate if the customers provide accurate and real info about their car models and driving history. Lying about past driving incidents can make the price estimates to be lower, but when dealing with an insurance company lying to them is useless. Usually, insurance companies will do research about a potential customer before granting him coverage. Online quotes can be sorted easily. Although drivers are recommended to not choose a policy just based on its price, drivers can easily sort quotes by insurance price. Using brokerage websites will allow drivers to get quotes from multiple insurers, thus making the comparison faster and easier. For additional info, money-saving tips, and free car insurance quotes, visit https://compare-autoinsurance.Org/ Compare-autoinsurance.Org is an online provider of life, home, health, and auto insurance quotes. This website is unique because it does not simply stick to one kind of insurance provider, but brings the clients the best deals from many different online insurance carriers. In this way, clients have access to offers from multiple carriers all in one place: this website. On this site, customers have access to quotes for insurance plans from various agencies, such as local or nationwide agencies, brand names insurance companies, etc. "Online quotes can easily help drivers obtain better car insurance deals. All they have to do is to complete an online form with accurate and real info, then compare prices", said Russell Rabichev, Marketing Director of Internet Marketing Company. CONTACT: Company Name: Internet Marketing CompanyPerson for contact Name: Gurgu CPhone Number: (818) 359-3898Email: [email protected]: https://compare-autoinsurance.Org/ SOURCE: Compare-autoinsurance.Org View source version on accesswire.Com:https://www.Accesswire.Com/595055/What-Are-The-Main-Benefits-Of-Comparing-Car-Insurance-Quotes-Online View photos
to request, modification Contact us at Here or [email protected]