Tech
4 innovative AI/ML techniques to transform your data pipeline
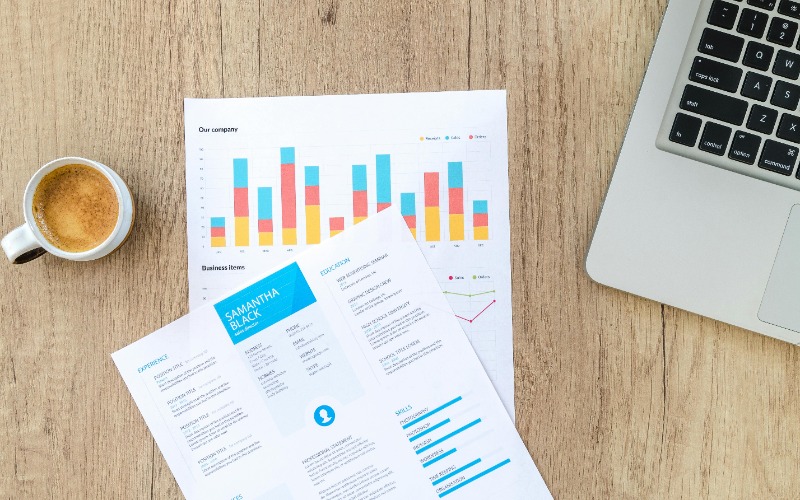
In today's AI and ML digital age, data pipelines are the foundation for managing and processing the vast amounts of data generated by modern businesses. These pipelines are critical for aggregating, organizing, and analyzing data from disparate sources, allowing businesses to derive actionable insights and make informed decisions. Efficient data pipelines allow data to flow smoothly from collection to consumption, supporting everything from real-time analytics to machine learning applications. As the volume, variety, and velocity of data continues to grow, optimizing these pipelines is essential to maintaining competitive advantage and operational efficiency.
Integrating artificial intelligence (AI) and machine learning (ML) into data pipelines is a major evolution in how companies process data. AI and ML power data pipelines by automating complex processes, improving the accuracy of data analysis, and enabling more effective decision-making. These technologies predict patterns, discover anomalies, and provide insights beyond the capabilities of traditional data processing methods. By adopting AI and ML, companies can not only streamline data operations, but also unlock new opportunities for innovation and growth, and remain agile in a rapidly changing business environment.
TrendingMichael Shannon talks about playing George Westinghouse on 'The Current War' Technique 1: Automatic data cleansing
Automated data cleansing powered by AI and ML is revolutionizing the way businesses process data by improving the quality and reliability of information flowing through the pipeline. This process involves using machine learning algorithms to systematically clean data to remove inaccuracies, inconsistencies, and duplication without human intervention. Using techniques such as anomaly detection and pattern recognition, these systems can identify outliers and errors that can compromise data integrity. By automating this critical step, organizations can ensure that their data is accurate and reliable. This is essential to the analysis and decision-making process.
Anomaly detection algorithms are particularly effective for automatic data cleansing. They work by establishing normal patterns in your data and flagging deviations from these patterns as potential anomalies that need to be investigated and corrected. Pattern recognition further assists this process by analyzing data for recurring structures and themes, helping to automate complex decisions about data validity and relevance. Together, these technologies minimize the risk of making data-driven decisions based on flawed data and improve operational efficiency.
A notable example of automatic data cleansing in action is IBM's use of Watson AI to improve data quality for business analytics. Watson employs advanced machine learning models to detect anomalies and patterns and cleanse data without human oversight. This application of AI to data cleansing has enabled IBM and its customers to significantly improve the efficiency of their data pipelines and ensure that the data used in decision-making processes is accurate and timely. . This provides more reliable business insights and better outcomes, demonstrating the practical benefits of integrating AI into your data management strategy.
Technique 2: Predictive analytics for data flow optimization
Predictive analytics is an innovative tool that optimizes data pipelines by predicting data loads and processing times with incredible accuracy. By leveraging AI-driven models, enterprises can proactively manage and streamline data workflows and use data resources efficiently. These predictive models analyze historical data patterns to predict future needs, allowing organizations to proactively scale up or down resources based on expected data volumes. This capability is essential for maintaining seamless operations, especially in environments where data ingestion fluctuates.
AI models are great at identifying potential bottlenecks in data pipelines before they cause disruption. These models can flag areas of concern in real-time by continuously monitoring data flows and comparing them to predictive benchmarks. This allows businesses to make instant adjustments to their pipelines, including reallocating resources and adjusting processes to maintain optimal throughput. Such real-time optimization is critical in high-stakes industries where data delays can lead to significant financial losses and operational inefficiencies.
A great example of predictive analytics in action is how Amazon uses machine learning to manage the flow of data across its vast e-commerce platform. Amazon employs advanced AI algorithms to predict customer demand, adjust inventory levels, and optimize the fulfillment process. This predictive approach not only ensures that Amazon can handle large data loads during peak periods such as Black Friday sales, but also improves overall system performance by efficiently allocating computational resources. As a result, Amazon maintains high service levels and customer satisfaction, demonstrating the significant impact of predictive analytics on resource allocation and system performance.
Technique 3: Natural language processing (NLP) for unstructured data
Incorporating unstructured data into data pipelines poses significant challenges, primarily due to its diverse formats and non-standardized content. Unstructured data such as text, audio, and video lacks the orderly format of traditional database entries, making it difficult to process and analyze using traditional data tools. This diversity requires advanced processing techniques to extract the available information and integrate it into data-driven decision-making processes. As businesses increasingly rely on insights from social media, customer reviews, and other text-heavy sources, the ability to efficiently manage unstructured data is critical.
Natural language processing (NLP) provides a powerful solution by allowing valuable information to be extracted from text-based data. NLP techniques such as sentiment analysis, entity recognition, and topic modeling can be used to analyze text to identify key themes, opinions, and relationships. For example, sentiment analysis can evaluate customer feedback to measure overall satisfaction, while entity recognition can help identify and categorize specific items or issues mentioned in text data. This capability allows organizations to transform raw text into structured, actionable insights that can be easily integrated into data pipelines.
A real-life example of NLP transforming data pipelines is Twitter's use of NLP to enhance user engagement and ad targeting. Twitter uses NLP to process vast amounts of tweets to detect trending topics, monitor public opinion, and categorize content to serve better ads. The integration of NLP will allow Twitter to serve more relevant content to users, allowing advertisers to more effectively reach their target users. The benefits of incorporating NLP into your data pipeline include improving user experience, increasing advertising revenue, and increasing your ability to respond quickly to changing public opinion and market trends.
Technique 4: Real-time data processing using machine learning models
The shift from batch processing to real-time data processing in data pipelines represents a major advancement in the way enterprises process and leverage data. Traditional batch processing requires data to be collected and processed in large periodic chunks, which can slow decision-making and response times. In contrast, real-time data processing allows for continuous ingestion and analysis of incoming data, enabling immediate action and insight. This migration is particularly advantageous in dynamic environments where timely data is critical to operational success.
Machine learning models are at the core of this transformation, providing the tools needed to analyze and make decisions on streaming data. These models can quickly identify patterns, anomalies, and trends in real time, making it easier to respond quickly to changing conditions. For example, in financial trading, machine learning models analyze streaming market data and trigger automated trades based on specific market conditions. This feature not only increases the responsiveness of your trading strategies, but also helps reduce risks associated with market volatility.
Real-time data processing is essential in industries such as financial services, where milliseconds can mean the difference between big profits and losses. It is also important in emergency response systems where real-time data can inform immediate actions to manage and mitigate crises. For example, earthquake early warning systems use real-time data processing to analyze seismic data and issue warnings seconds before shaking begins, potentially saving lives and reducing damage. These applications highlight the transformative impact of integrating machine learning models into real-time data processing and demonstrate their critical role in modern data-driven operations.
Embrace the future of data management
The integration of AI and ML into data pipelines is not just a trend, but a paradigm shift that will define the future of data management. Organizations that embrace these advanced technologies are setting new standards for efficiency and insight, paving the way for innovations once thought impossible. As we move forward, the convergence of real-time processing, predictive analytics, and automated systems will continue to break down barriers and provide unprecedented opportunities for those ready to embrace these changes. The future belongs to businesses that leverage AI and ML to turn real-time data into real-time decisions.
Sources 2/ https://innotechtoday.com/4-innovative-ai-ml-techniques-transforming-data-pipelines/ The mention sources can contact us to remove/changing this article |
What Are The Main Benefits Of Comparing Car Insurance Quotes Online
LOS ANGELES, CA / ACCESSWIRE / June 24, 2020, / Compare-autoinsurance.Org has launched a new blog post that presents the main benefits of comparing multiple car insurance quotes. For more info and free online quotes, please visit https://compare-autoinsurance.Org/the-advantages-of-comparing-prices-with-car-insurance-quotes-online/ The modern society has numerous technological advantages. One important advantage is the speed at which information is sent and received. With the help of the internet, the shopping habits of many persons have drastically changed. The car insurance industry hasn't remained untouched by these changes. On the internet, drivers can compare insurance prices and find out which sellers have the best offers. View photos The advantages of comparing online car insurance quotes are the following: Online quotes can be obtained from anywhere and at any time. Unlike physical insurance agencies, websites don't have a specific schedule and they are available at any time. Drivers that have busy working schedules, can compare quotes from anywhere and at any time, even at midnight. Multiple choices. Almost all insurance providers, no matter if they are well-known brands or just local insurers, have an online presence. Online quotes will allow policyholders the chance to discover multiple insurance companies and check their prices. Drivers are no longer required to get quotes from just a few known insurance companies. Also, local and regional insurers can provide lower insurance rates for the same services. Accurate insurance estimates. Online quotes can only be accurate if the customers provide accurate and real info about their car models and driving history. Lying about past driving incidents can make the price estimates to be lower, but when dealing with an insurance company lying to them is useless. Usually, insurance companies will do research about a potential customer before granting him coverage. Online quotes can be sorted easily. Although drivers are recommended to not choose a policy just based on its price, drivers can easily sort quotes by insurance price. Using brokerage websites will allow drivers to get quotes from multiple insurers, thus making the comparison faster and easier. For additional info, money-saving tips, and free car insurance quotes, visit https://compare-autoinsurance.Org/ Compare-autoinsurance.Org is an online provider of life, home, health, and auto insurance quotes. This website is unique because it does not simply stick to one kind of insurance provider, but brings the clients the best deals from many different online insurance carriers. In this way, clients have access to offers from multiple carriers all in one place: this website. On this site, customers have access to quotes for insurance plans from various agencies, such as local or nationwide agencies, brand names insurance companies, etc. "Online quotes can easily help drivers obtain better car insurance deals. All they have to do is to complete an online form with accurate and real info, then compare prices", said Russell Rabichev, Marketing Director of Internet Marketing Company. CONTACT: Company Name: Internet Marketing CompanyPerson for contact Name: Gurgu CPhone Number: (818) 359-3898Email: [email protected]: https://compare-autoinsurance.Org/ SOURCE: Compare-autoinsurance.Org View source version on accesswire.Com:https://www.Accesswire.Com/595055/What-Are-The-Main-Benefits-Of-Comparing-Car-Insurance-Quotes-Online View photos
to request, modification Contact us at Here or [email protected]