Health
Revolutionary AI tool forecasts kidney injury signs with high accuracy in intensive care
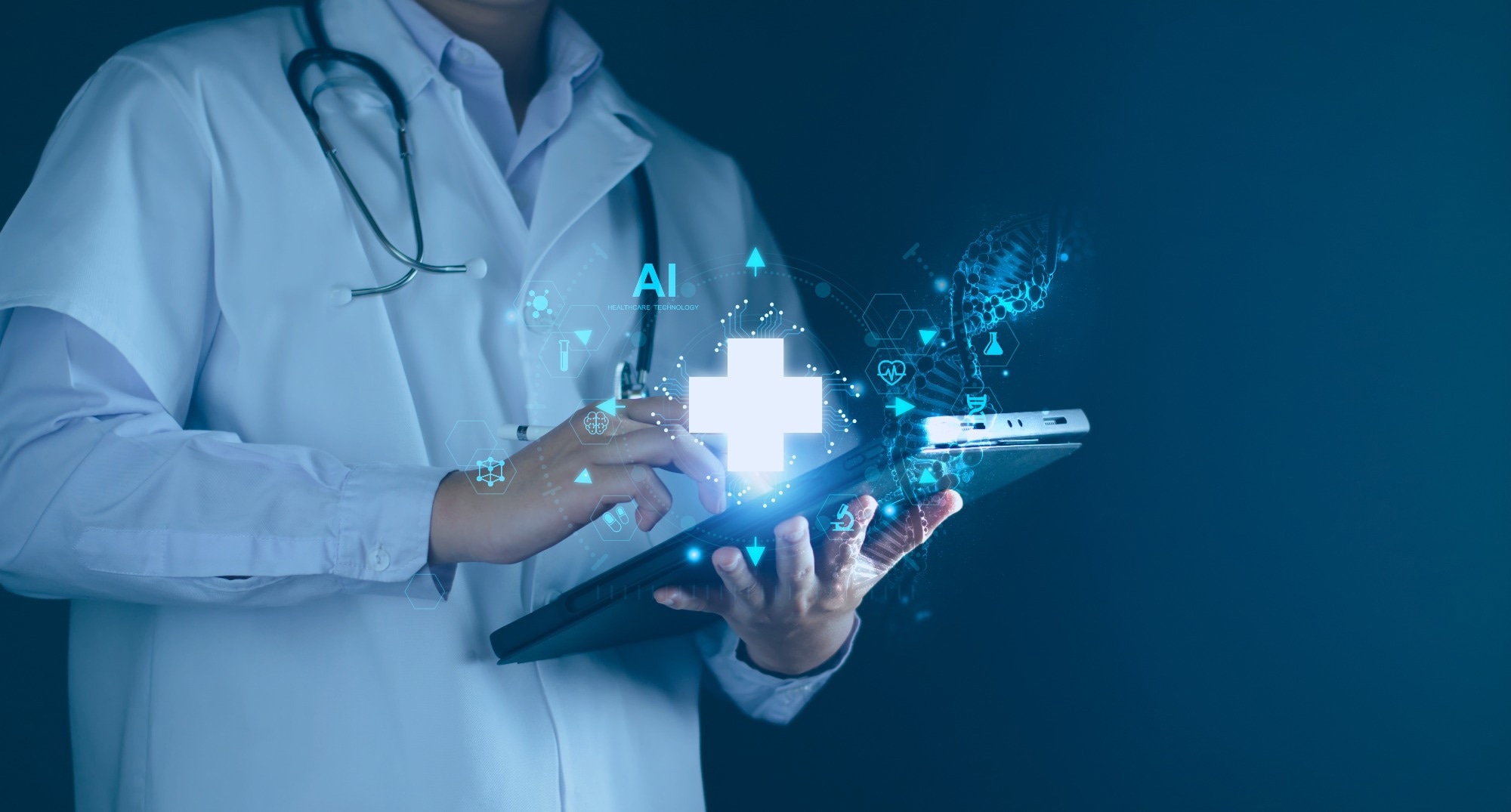
In a recent study published in Scientific Reports, researchers developed a machine-learning (ML) algorithm for predicting oliguria.
They validated its accuracy in a large cohort of patients admitted to medical or surgical intensive care units (ICUs) at Chiba University Hospital in Japan between November 2010 and March 2019.
Study: Machine-learning model for predicting oliguria in critically ill patients. Image Credit: LALAKA/Shutterstock.com
Background
Oliguria, i.e., a urine output of <0.5 mL/kg/h, is a measure that can predict acute kidney injury (AKI) in ICU patients.
AKI, characterized by a swift surge in serum creatinine levels or declines in urine output, is a leading cause of complications during ICU admissions, which, at its worst, can even result in organ dysfunction and death.
Its early detection and prompt intervention(s) can improve the prognosis of severely ill ICU patients, further showing diagnostic implications for oliguria in AKI management.
Studies have evaluated ML approaches for predicting urine output in critically ill patients; however, their precision in predicting oliguria in an ICU setting remains unknown.
About the study
In the present study, researchers used an ML algorithm to predict oliguria in critically ill patients, occurring at 6 and 72 hours, starting from an arbitrary time during their ICU stay.
They defined AKI as a serum creatinine level of at least 0.3 mg/dL above baseline and oliguria as urine output of less than 0.5 mL/kg/h.
The patient electronic health records (EHRs), i.e., the initial study dataset, encompassed 1,031 input variables, such as their physiological measurements, blood tests, medications, general observation and care records, and blood transfusion details.
The team calculated hourly time-series tables of patients’ urine and stool volumes (physiological measurements) and used the most recent test values used for blood tests.
Using these hourly variables and baseline information, the team developed an ML model to predict oliguria in ICU patients.
In this, they added 222 background information variables, e.g., age, which resulted in 1,127 variables in the final dataset.
Further, they removed potential collinearity values and treated missing values separately or excluded them from the analysis.
The team validated the performance of four ML classifiers using a fivefold cross-validation, where the calibration curve indicated that the model was well-calibrated.
LightGBM prediction model showed the best speed and area under the curve (AUC). The AUC values ranged from zero to one, with one indicating a perfect prediction.
Furthermore, Shapley additive explanation (SHAP) values helped evaluate key variables, improving the interpretability of ML models.
SHAP individual force plots helped visualize the impact of different variables on the probability of oliguria occurrence, further enhancing the understanding of the oliguria occurrence in two patients, one with oliguria and one without oliguria, based on their traits.
Results
Of 9,241 ICU patients included in the study, 27.4% and 30.2% had urine output <0.5 mL/kg/h for six hours and AKI, respectively. The ML model showed an AUC of 0.964 to predict oliguria at six hours using certain variables.
The cross-validation resulted in a slightly lower AUC of 0.920, but close to the original AUC, suggesting the model’s high accuracy in predicting oliguria at six hours.
SHAP values found to be important for predicting oliguria at six hours were sequential organ failure assessment (SOFA) score, urine values, serum creatinine levels, creatinine kinase, total bilirubin, oxygen partial pressure (pO2), interleukin (IL)-6, fibrinogen/fibrin degradation products (FDP), and peripheral temperature. These variables also well-predicted oliguria at 72 hours.
When comparing two patients with different traits, SHAP analysis suggested that a higher urine volume reduced the probability of oliguria occurrence, and increased lactate dehydrogenase (LDH) & FDP levels increased this probability.
Therefore, the model predicted a lower likelihood of oliguria occurrence at six hours for the first patient.
In the second patient, a higher acute physiology and chronic health evaluation (APACHE) II score and lower urine volume increased the probability of oliguria, and normal uric acid (UA), LDH, and IL-6 levels reduced it. The model, thus, predicted an increased oliguria risk at six hours for this patient.
The AUC of the prediction model for oliguria at 72 hours was 0.916, yet again, suggesting its high accuracy.
In the subgroup analyses, the accuracy of the model in the female and furosemide groups declined over time than in the other groups, with relatively high accuracy (AUC = 0.86) even at 72 h.
Conclusions
According to the authors, previous studies have verified the precision of an ML model to predict AKI in critically ill patients but with lower accuracies, AUCs in the range of 0.74 to 0.93.
The current study model, on the contrary, showed higher accuracy (> 0.90), which they attributed to the large sample size and choosing a method of predicting the onset of oliguria from an arbitrary time, which resulted in more training datasets.
Because oliguria could identify more patients with AKI earlier than serum creatinine levels-based diagnosis, the study model could help with early detection of patients with AKI, improving the prognosis of this population manifold through better management and early interventions.
Future work can focus on validating the accuracy of ML-based prediction models further.
Journal reference:
-
Yamao, Y., Oami, T., Yamabe, J., Takahashi, N., & Nakada, T. (2024). Machine-learning model for predicting oliguria in critically ill patients. Scientific Reports, 14(1), 1-9. doi: https://doi.org/10.1038/s41598-024-51476-y. https://www.nature.com/articles/s41598-024-51476-y
Sources 2/ https://www.news-medical.net/news/20240115/Revolutionary-AI-tool-forecasts-kidney-injury-signs-with-high-accuracy-in-intensive-care.aspx The mention sources can contact us to remove/changing this article |
What Are The Main Benefits Of Comparing Car Insurance Quotes Online
LOS ANGELES, CA / ACCESSWIRE / June 24, 2020, / Compare-autoinsurance.Org has launched a new blog post that presents the main benefits of comparing multiple car insurance quotes. For more info and free online quotes, please visit https://compare-autoinsurance.Org/the-advantages-of-comparing-prices-with-car-insurance-quotes-online/ The modern society has numerous technological advantages. One important advantage is the speed at which information is sent and received. With the help of the internet, the shopping habits of many persons have drastically changed. The car insurance industry hasn't remained untouched by these changes. On the internet, drivers can compare insurance prices and find out which sellers have the best offers. View photos The advantages of comparing online car insurance quotes are the following: Online quotes can be obtained from anywhere and at any time. Unlike physical insurance agencies, websites don't have a specific schedule and they are available at any time. Drivers that have busy working schedules, can compare quotes from anywhere and at any time, even at midnight. Multiple choices. Almost all insurance providers, no matter if they are well-known brands or just local insurers, have an online presence. Online quotes will allow policyholders the chance to discover multiple insurance companies and check their prices. Drivers are no longer required to get quotes from just a few known insurance companies. Also, local and regional insurers can provide lower insurance rates for the same services. Accurate insurance estimates. Online quotes can only be accurate if the customers provide accurate and real info about their car models and driving history. Lying about past driving incidents can make the price estimates to be lower, but when dealing with an insurance company lying to them is useless. Usually, insurance companies will do research about a potential customer before granting him coverage. Online quotes can be sorted easily. Although drivers are recommended to not choose a policy just based on its price, drivers can easily sort quotes by insurance price. Using brokerage websites will allow drivers to get quotes from multiple insurers, thus making the comparison faster and easier. For additional info, money-saving tips, and free car insurance quotes, visit https://compare-autoinsurance.Org/ Compare-autoinsurance.Org is an online provider of life, home, health, and auto insurance quotes. This website is unique because it does not simply stick to one kind of insurance provider, but brings the clients the best deals from many different online insurance carriers. In this way, clients have access to offers from multiple carriers all in one place: this website. On this site, customers have access to quotes for insurance plans from various agencies, such as local or nationwide agencies, brand names insurance companies, etc. "Online quotes can easily help drivers obtain better car insurance deals. All they have to do is to complete an online form with accurate and real info, then compare prices", said Russell Rabichev, Marketing Director of Internet Marketing Company. CONTACT: Company Name: Internet Marketing CompanyPerson for contact Name: Gurgu CPhone Number: (818) 359-3898Email: [email protected]: https://compare-autoinsurance.Org/ SOURCE: Compare-autoinsurance.Org View source version on accesswire.Com:https://www.Accesswire.Com/595055/What-Are-The-Main-Benefits-Of-Comparing-Car-Insurance-Quotes-Online View photos
to request, modification Contact us at Here or [email protected]